Importance Driven Visualization of Molecular Surfaces
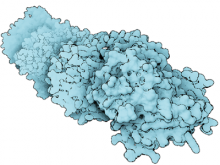
We present a novel rendering method, based on the level-of-detail concept, which shows protein complexes over time in real-time. We exploit three different molecular surface models, solvent excluded surface (SES), Gaussian kernels and van der Waals spheres combined in one seamless visualization. As a general rule, closest to the viewer we aim at providing a maximum of relevant information related to the structure and binding sites. Such information is conveyed by the SES representation. Farther away from the viewer, we are smoothly changing the visual representation to an approximation of SES through Gaussian kernels. The least detailed representation is based on simple sphere splatting and is dedicated to structures farthest away from the viewer. A more general solution leads us to the definition of a 3D importance function that is based on the distance measure from a molecular feature. In order to preserve smoothness in transition areas, we introduce three shading levels that correspond to their geometric counterparts and a method for creating seamless transition between these representations. The SES representation with full shading and added contours stands in focus while on the other side a sphere representation with constant shading and without contours provide the context. Moreover, we introduce a methodology to render the entire molecule directly using the A-buffer technique, which further improves the performance. The rendering performance is evaluated on series of molecules of varying atom counts.
BioVis 2013 Information
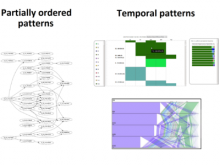
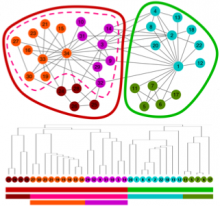
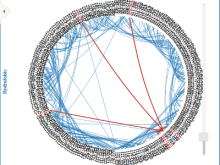
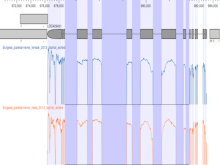
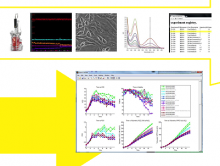
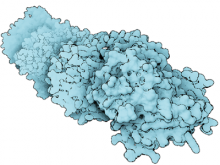
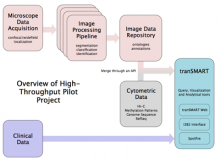
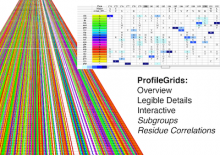
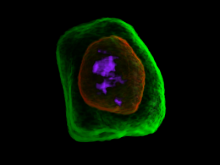
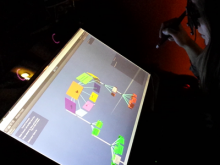
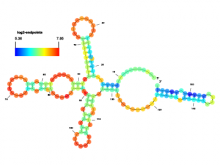
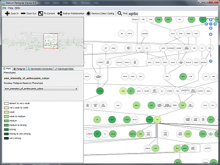
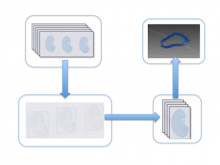
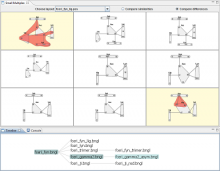
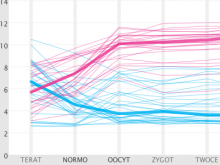
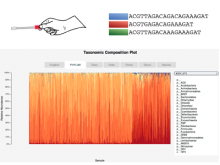
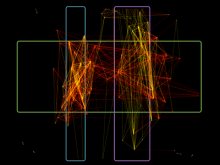
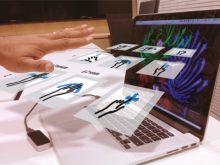
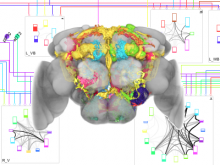
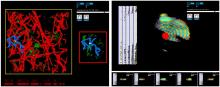

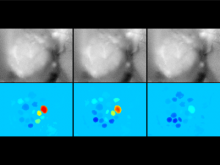
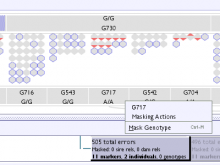
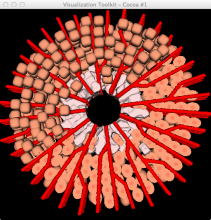
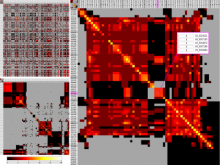
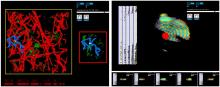
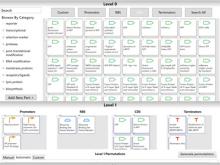
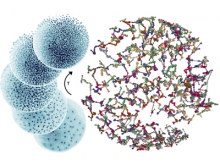
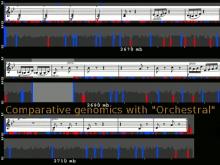
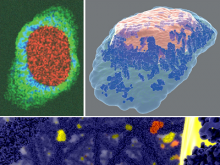
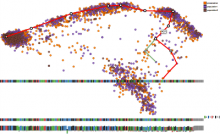